Retailer-level recommendations for one of the largest food and beverage company
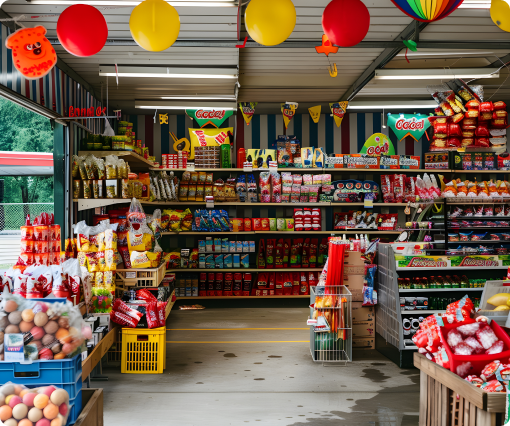
Overview
Our client is one of the world’s largest food and beverage companies and wanted to build a retailer recommendation at a retailer X product level by unlocking key patterns in the historical purchase orders.
The objectives of our engagement included developing a complex deep learning-based recommendation engine to recommend products for each retailer in a particular area for “Must Sell” and “Cross Sell” categories. The engine must handle large data and could be scaled to more regions to improve “range sales” and penetration across product segments incl. new segments such as “Ready-to-eat”.
Solution
- Filtered all master data such as ‘Retailer’, ‘Product’, ‘Distributor’ based on transactional data
- Products and retailers with only zero or negative transactions were excluded, transactional data was aggregated monthly, and retailer channels contributing a minor percentage of sales and product categories were all grouped under “Others”.
- Retailers were segmented based on recency, frequency, and monetary values (RFM), while products were segmented by revenue and quantity sold, categorizing them as core, high, medium, or low products.
- Product-based recommendations for retailers were implemented using collaborative filtering with machine learning models such as Neural Collaborative Filtering, incorporating retailer features (e.g., retailer type, channel, recency, frequency) and product features (e.g., product category, brand, variant, grammage)
- Data Granularity
- Two years of transactional data
- The master data includes approx. 15,000 retailers, for over 500 products, and 20+ distributors.
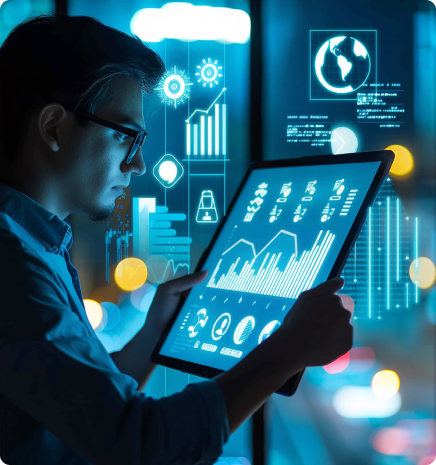
Impact
The model refreshes at the end of every month as recommendations should be rolled out to the salesperson at the start of the subsequent month.
TransOrg delivered a recommendation engine that recommends products for both ‘must sell’ and ‘cross sell’ at a retailer x product level incorporating inventory constraints.